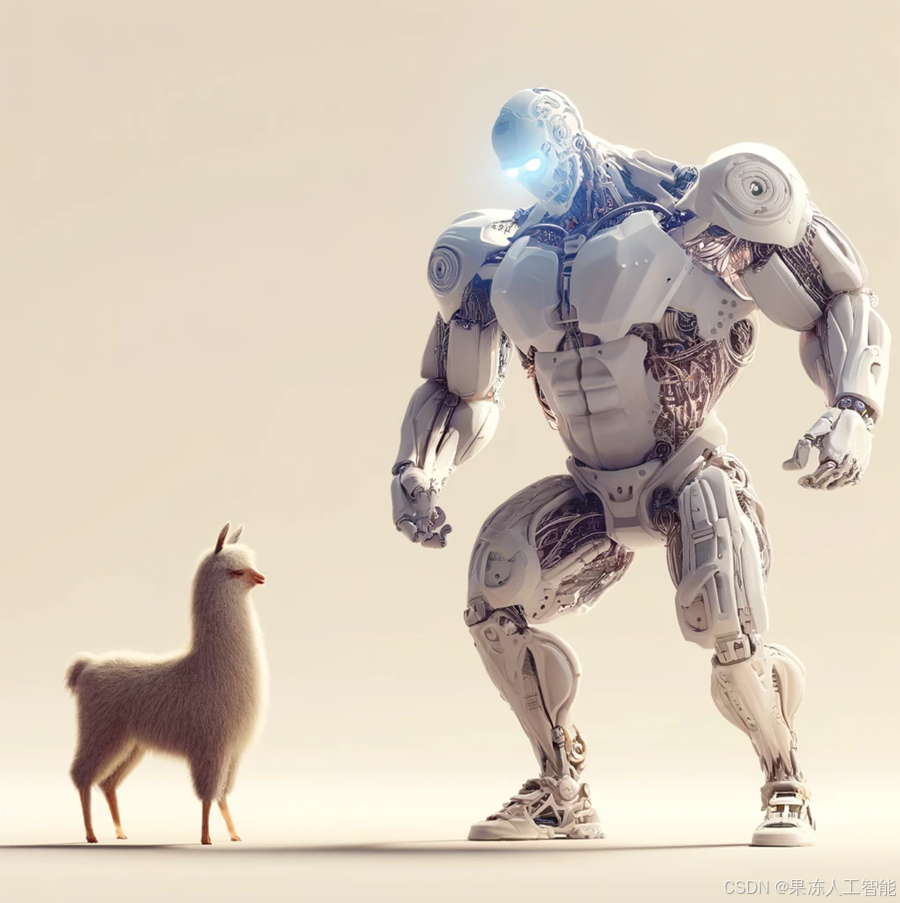
别忘了关注作者,关注后您会变得更聪明,不关注就只能靠颜值了 ^_^。
一位年轻的儿科医生与一位经验丰富的医师,谁更能有效治疗婴儿的咳嗽?
两者都具备治疗咳嗽的能力,但儿科医生由于专攻儿童医学,或许在诊断婴儿疾病方面更具优势。这也正如小模型在某些特定任务上的表现,往往经过微调后能够比大型模型更为出色,尽管大型模型号称可以处理任何问题。
最近,我面临了一个必须在两者之间做出选择的场景。
我正在开发一个查询路由系统,用于将用户的请求引导至合适的部门,然后由人工继续对话。从技术角度看,这是一个文本分类任务。虽然GPT-4o及其小版本在这类任务上表现优秀,但它的使用成本较高,且由于是封闭模型,我无法在自己的环境中进行微调。尽管OpenAI提供了微调服务,但对我来说,成本仍然过于昂贵。
每百万个Token的训练费用为25美元,而我的训练数据量很快就达到了数百万个Token。再加上微调后的模型使用费用比普通模型高50%,这对我的小型项目而言,预算无疑是无法承受的。因此,我必须寻找一个替代方案。
相比之下,开源模型在处理分类任务时同样表现不俗,且训练成本相对较低,尤其是在使用GPU时。经过慎重考虑,我决定转向小型模型。小型LLM通过微调可以在有限的预算下实现令人满意的效果,这是我目前最为理想的选择。
小型模型可以在普通硬件上运行,微调所需的GPU也不必过于昂贵。更为重要的是,小模型的训练和推理速度远快于大型LLM。
经过一番调研,我挑选了几款候选模型——Phi3.5、DistillBERT和GPT-Neo,但最终选择了Meta Llama 3.2的1B模型。这个选择并非完全理性,部分原因可能是最近关于这个模型的讨论较多。不过,实践出真知,我决定通过实测来检验效果。
在接下来的部分,我将分享我微调Llama 3.2–1B指令模型与使用少样本提示的GPT-4o的对比结果。
微调Llama 3.2 1B模型(免费实现微调)
微调模型的确可能需要较高的成本,但如果选择合适的策略,还是能够大幅降低开支。针对我的情况,我采用了参数优化的微调(PEFT)策略,而不是完全参数微调。完全微调会重新训练模型中的全部1B参数,成本太高,且可能导致“灾难性遗忘”,即模型丢失预训练时学到的部分知识。而PEFT策略则聚焦于仅微调部分参数,大大减少了时间和资源的消耗。
其中,“低秩适应”(LORA)技术是目前较为流行的微调方法。LORA允许我们仅对某些特定层的部分参数进行微调,这样的训练不仅高效且效果明显。
此外,通过模型量化,我们可以将模型的参数压缩为float16甚至更小的格式,这不仅减少了内存消耗,还能提高计算速度。当然,精度可能会有所下降,但对于我的任务来说,这一折衷是可以接受的。
接下来,我将在免费的Colab和Kaggle平台上进行了微调。这些平台提供的GPU资源虽然有限,但对于像我这样的小模型训练任务已经足够,关键它们免费。
Llama-3.2微调与GPT-4o少样本提示的对比
微调Llama 3.2 1B模型的过程相对简单。我参考了Unsloth提供的Colab笔记本,并做了部分修改。原笔记本微调的是3B参数的模型,而我将其改为1B参数的Llama-3.2–Instruct,因为我想测试较小模型在分类任务上的表现。接着,我将数据集替换为我自己的数据,用于训练。
# Beforefrom unsloth.chat_templates import standardize_sharegptdataset = standardize_sharegpt(dataset)dataset = dataset.map(formatting_prompts_func, batched = True,)# Afterfrom datasets import Datasetdataset = Dataset.from_json("/content/insurance_training_data.json")dataset = dataset.map(formatting_prompts_func, batched = True,)
最稳妥的做法是选择一个与笔记本初始设计相符的数据集,例如下面的这个。
{"conversations": [{'role': 'user', 'content': <user_query>}{'role': 'assistant', 'content': <department>}]}
到这里为止,这两处调整已经足够让你用自己的数据微调模型了。
评估微调后的模型
接下来是关键的一步:评估测试。
评估LLM是一项广泛且富有挑战性的工作,也是LLM开发中最为重要的技能之一。我将再出一篇文章,在其中详细讨论过如何评估LLM应用,别忘了关注作者,关注后您会变得更聪明,不关注就只能靠颜值了 ^_^。
不过,为了简洁起见,这次我会采用经典的混淆矩阵方式进行评估。只需在笔记本的末尾添加下面的代码即可。
from langchain.prompts import FewShotPromptTemplatefrom langchain_openai import ChatOpenAIfrom langchain_core.prompts import PromptTemplatefrom pydantic import BaseModel# 1. A function to generate response with the fine-tuned modeldef generate_response(user_query):# Enable faster inference for the language modelFastLanguageModel.for_inference(model)# Define the message templatemessages = [{"role": "system", "content": "You are a helpful assistant who can route the following query to the relevant department."},{"role": "user", "content": user_query},]# Apply the chat template to tokenize the input and prepare for generationtokenized_input = tokenizer.apply_chat_template(messages,tokenize=True,add_generation_prompt=True, # Required for text generationreturn_tensors="pt").to("cuda") # Send input to the GPU# Generate a response using the modelgenerated_output = model.generate(input_ids=tokenized_input,max_new_tokens=64,use_cache=True, # Enable cache for faster generationtemperature=1.5,min_p=0.1)# Decode the generated tokens into human-readable textdecoded_response = tokenizer.batch_decode(generated_output, skip_special_tokens=True)[0]# Extract the assistant's response (after system/user text)assistant_response = decoded_response.split("\n\n")[-1]return assistant_response# 2. Generate Responeses with OpenAI GPT-4o# Define the prompt template for the exampleexample_prompt_template = PromptTemplate.from_template("User Query: {user_query}\n{department}")# Initialize OpenAI LLM (ensure the OPENAI_API_KEY environment variable is set)llm = ChatOpenAI(temperature=0, model="gpt-4o")# Define few-shot examplesexamples = [{"user_query": "I recently had an accident and need to file a claim for my vehicle. Can you guide me through the process?", "department": "Claims"},...]# Create a few-shot prompt templatefew_shot_prompt_template = FewShotPromptTemplate(examples=examples,example_prompt=example_prompt_template,prefix="You are an intelligent assistant for an insurance company. Your task is to route customer queries to the appropriate department.",suffix="User Query: {user_query}",input_variables=["user_query"])# Define the department model to structure the outputclass Department(BaseModel):department: str# Function to predict the appropriate department based on user querydef predict_department(user_query):# Wrap LLM with structured outputstructured_llm = llm.with_structured_output(Department)# Create the chain for generating predictionsprediction_chain = few_shot_prompt_template | structured_llm# Invoke the chain with the user query to get the departmentresult = prediction_chain.invoke(user_query)return result.department# 3. Read your evaluation dataset and predict departmentsimport jsonwith open("/content/insurance_bot_evaluation_data (1).json", "r") as f:eval_data = json.load(f)for ix, item in enumerate(eval_data):print(f"{ix+1} of {len(eval_data)}")item['open_ai_response'] = generate_response(item['user_query'])item['llama_response'] = item['open_ai_response']# 4. Compute the precision, recall, accuracy, and F1 scores for the predictions.# 4.1 Using Open AIfrom sklearn.metrics import precision_score, recall_score, accuracy_score, f1_scoretrue_labels = [item['department'] for item in eval_data]predicted_labels_openai = [item['open_ai_response'] for item in eval_data]# Calculate the scores for open_ai_responseprecision_openai = precision_score(true_labels, predicted_labels_openai, average='weighted')recall_openai = recall_score(true_labels, predicted_labels_openai, average='weighted')accuracy_openai = accuracy_score(true_labels, predicted_labels_openai)f1_openai = f1_score(true_labels, predicted_labels_openai, average='weighted')print("OpenAI Response Scores:")print("Precision:", precision_openai)print("Recall:", recall_openai)print("Accuracy:", accuracy_openai)print("F1 Score:", f1_openai)# 4.2 Using Fine-tuned Llama 3.2 1B Instructtrue_labels = [item['department'] for item in eval_data]predicted_labels_llama = [item['llama_response'] for item in eval_data]# Calculate the scores for llama_responseprecision_llama = precision_score(true_labels, predicted_labels_llama, average='weighted', zero_division=0)recall_llama = recall_score(true_labels, predicted_labels_llama, average='weighted', zero_division=0)accuracy_llama = accuracy_score(true_labels, predicted_labels_llama)f1_llama = f1_score(true_labels, predicted_labels_llama, average='weighted', zero_division=0)print("Llama Response Scores:")print("Precision:", precision_llama)print("Recall:", recall_llama)print("Accuracy:", accuracy_llama)print("F1 Score:", f1_llama)
以上代码非常清晰明了。我们编写了一个函数,利用微调后的模型进行部门预测。同时,也为OpenAI GPT-4o构建了一个类似的函数。
接着,我们使用这些函数对评估数据集生成预测结果。
评估数据集中包含了预期的分类,现在我们也获得了模型生成的分类,这为接下来的指标计算提供了基础。
接下来,我们将进行这些计算。
以下是结果:
OpenAI Response Scores:Precision: 0.9Recall: 0.75Accuracy: 0.75F1 Score: 0.818Llama Response Scores:Precision: 0.88Recall: 0.73Accuracy: 0.79F1 Score: 0.798
结果显示,微调后的模型表现几乎接近GPT-4o。对于一个只有1B参数的小型模型来说,这已经相当令人满意了。
尽管GPT-4o的表现确实更好,但差距非常微小。
此外,如果在少样本提示中提供更多示例,GPT-4o的结果可能会进一步提升。不过,由于我的示例有时比较长,甚至包括几段文字,这会显著增加成本,毕竟OpenAI是按输入Token计费的。
总结
我现在对小型LLM非常认可。它们运行速度快,成本低,而且在大多数使用场景中都能满足需求,尤其是在不进行微调的情况下。
在这篇文章中,我讨论了如何微调Llama 3.2 1B模型。该模型可以在较为普通的硬件上运行,而且微调成本几乎为零。我当前的任务是文本分类。
当然,这并不意味着小型模型能够全面超越像GPT-4o这样的巨型模型,甚至也不一定能胜过Meta Llama的8B、11B或90B参数的模型。较大的模型拥有更强的多语言理解能力、视觉指令处理能力,以及更加广泛的世界知识。
我的看法是,如果这些“超级能力”不是你当前的需求,为什么不选择一个小型LLM呢?”