旅游集市数仓建设
文章目录
- 旅游集市数仓建设
- 为什么要设计数据分层?
- 分层设计
- ODS(Operational Data Store):数据运营层
- DW(Data Warehouse):数据仓库层
- DWD(Data Warehouse Detail):数据明细层
- DWM(Data Warehouse Middle):数据中间层
- DWS(Data Warehouse Service):数据服务层
- ADS/APP/DM(Application Data Store/Application/DataMarket):数据应用层/数据集市
- DIM(Dimension):维表层
- 可能会用到的一些UDF
- 添加资源并注册函数
- get_points
- dateBetweenUDF
- calLength
- get_city_or_prov_id
- 涉及到的一些表:
- ODS层
- ods_oidd
- ods_wcdr
- ods_ddr
- ods_dpi
- DWD层:
- dwd_res_regn_mergelocation_msk_d
- mergeSQL
- dwm_staypoint_msk_d
- SQL
- dws_province_tourist_msk_d
- dws_city_tourist_msk_d
- dws_county_tourist_msk_d
- SQL
- DIM层
- dim_usertag_msk_m
- ADS层
- 需求矩阵
- 根据省游客表计算如下指标
- 根据市游客表计算如下指标
- 根据区县游客表计算如下指标
为什么要设计数据分层?
作为一名数据的规划者,我们肯定希望自己的数据能够有秩序地流转,数据的整个生命周期能够清晰明确被设计者和使用者感知到。直观来讲就是如下的左图这般层次清晰、依赖关系直观。
但是,大多数情况下,我们完成的数据体系却是依赖复杂、层级混乱的。如下的右图,在不知不觉的情况下,我们可能会做出一套表依赖结构混乱,甚至出现循环依赖的数据体系。
因此,我们需要一套行之有效的数据组织和管理方法来让我们的数据体系更有序,这就是谈到的数据分层。数据分层并不能解决所有的数据问题,但是,数据分层却可以给我们带来如下的好处:
-
清晰数据结构:每一个数据分层都有它的作用域和职责,在使用表的时候能更方便地定位和理解
-
减少重复开发:规范数据分层,开发一些通用的中间层数据,能够减少极大的重复计算
-
统一数据口径:通过数据分层,提供统一的数据出口,统一对外输出的数据口径
-
复杂问题简单化:将一个复杂的任务分解成多个步骤来完成,每一层解决特定的问题
分层设计
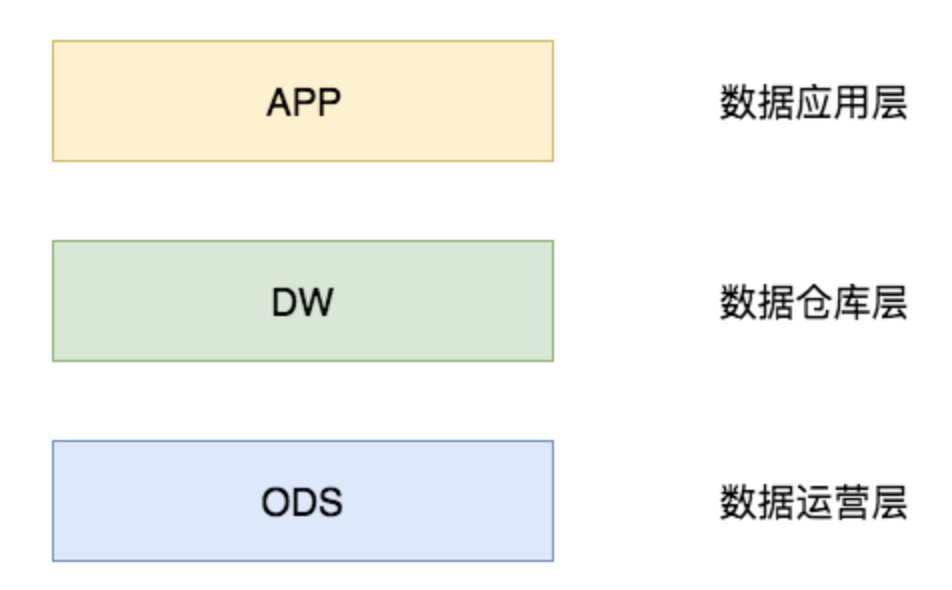
ODS(Operational Data Store):数据运营层
“面向主题的”数据运营层,也叫ODS层,是最接近数据源中数据的一层,数据源中的数据,经过抽取、洗净、传输,也就说传说中的 ETL 之后,装入本层。本层的数据,总体上大多是按照源头业务系统的分类方式而分类的。
一般来讲,为了考虑后续可能需要追溯数据问题,因此对于这一层就不建议做过多的数据清洗工作,原封不动地接入原始数据即可,至于数据的去噪、去重、异常值处理等过程可以放在后面的DWD层来做。
DW(Data Warehouse):数据仓库层
数据仓库层是我们在做数据仓库时要核心设计的一层,在这里,从 ODS 层中获得的数据按照主题建立各种数据模型。DW层又细分为 DWD(Data Warehouse Detail)层、DWM(Data WareHouse Middle)层和DWS(Data Warehouse Service)层。
该层一般保持和ODS层一样的数据粒度,并且提供一定的数据质量保证。同时,为了提高数据明细层的易用性,该层会采用一些维度退化手法,将维度退化至事实表中,减少事实表和维表的关联。
该层会在DWD层的数据基础上,对数据做轻度的聚合操作,生成一系列的中间表,提升公共指标的复用性,减少重复加工。直观来讲,就是对通用的核心维度进行聚合操作,算出相应的统计指标。
又称宽表。按照业务划分,如流量、订单、用户等,生成字段比较多的宽表,用于提供后续的业务查询,OLAP分析,数据分发等。
一般来讲,该层的数据表会相对比较少,一张表会涵盖比较多的业务内容,由于其字段较多,因此一般也会称该层的表为宽表。在实际计算中,如果直接从DWD或者ODS计算出宽表的统计指标,会存在计算量太大并且维度太少的问题,因此一般的做法是,在DWM层先计算出多个小的中间表,然后再拼接成一张DWS的宽表。由于宽和窄的界限不易界定,也可以去掉DWM这一层,只留DWS层,将所有的数据在放在DWS亦可。
ADS/APP/DM(Application Data Store/Application/DataMarket):数据应用层/数据集市
在这里,主要是提供给数据产品和数据分析使用的数据,一般会存放在 ES、PostgreSql、Redis等系统中供线上系统使用,也可能会存在 Hive 或者 Druid 中供数据分析和数据挖掘使用。比如我们经常说的报表数据,一般就放在这里。
DIM(Dimension):维表层
维表层主要包含两部分数据:
-
高基数维度数据:一般是用户资料表、商品资料表类似的资料表。数据量可能是千万级或者上亿级别。
-
低基数维度数据:一般是配置表,比如枚举值对应的中文含义,或者日期维表。数据量可能是个位数或者几千几万。
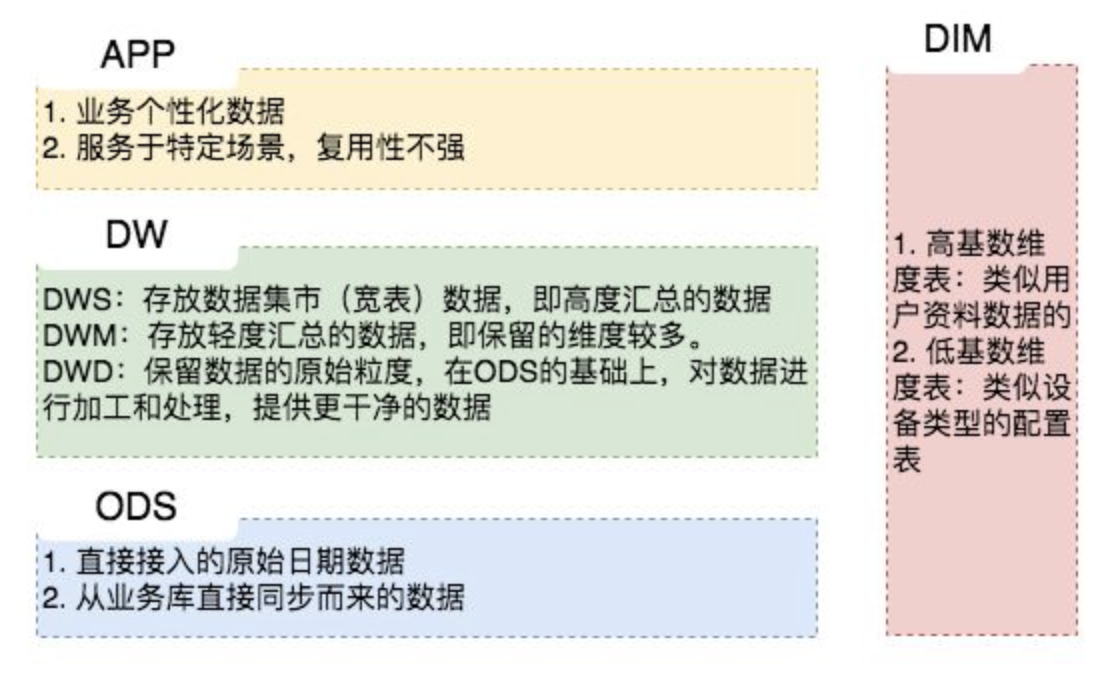
可能会用到的一些UDF
添加资源并注册函数
add jars /root/hive-1.0.jar;
create temporary function get_points as 'ctyun.udf.getPointsUDF';
create temporary function dateBetweenUDF as 'ctyun.udf.dateBetweenUDF';
create temporary function calLength as 'ctyun.udf.calLength';
create temporary function get_city_or_prov_id as 'ctyun.udf.getCityIdOrProvID';
get_points
传入网格id:grid_id,返回网格中心的经度、纬度
示例:select get_points(“845040”)[0] as longitude,get_points(“845040”)[1] as latitude;
package ctyun.udf;import ctyun.udf.grld.Grid;
import org.apache.hadoop.hive.ql.exec.UDF;import java.awt.geom.Point2D;
import java.lang.reflect.Array;
import java.util.ArrayList;public class getPointsUDF extends UDF {public ArrayList<Double> evaluate(String grid_id) {// 根据网格id 获取经纬度ArrayList<Double> cols = new ArrayList<Double>();Point2D.Double points = Grid.getCenter(Long.valueOf(grid_id));cols.add(points.x);cols.add(points.y);return cols;}
}
dateBetweenUDF
传入两个时间,返回时间差,单位:分
示例:select dateBetweenUDF(“20180503174500”, “20180503174000”);
package ctyun.udf;import ctyun.udf.util.DateUtil;
import org.apache.hadoop.hive.ql.exec.UDF;public class dateBetweenUDF extends UDF {public int evaluate(String grid_first_time,String grid_last_time) {// 获取两个时间字符串的差 单位:分return Math.abs(DateUtil.betweenM(grid_first_time,grid_last_time));}
}
calLength
传入两个网格id:grid_id,返回两个网格中心点的距离,单位:米
示例:select calLength(“845040”,“855040”);
package ctyun.udf;import ctyun.udf.util.Geography;
import org.apache.hadoop.hive.ql.exec.UDF;public class calLength extends UDF {public String evaluate(String grid_id, String resi_grid_id) {// 根据grid_id 网格id, resi_grid_id 居住地网格id 计算距离double distance = Geography.calculateLength(Long.valueOf(grid_id), Long.valueOf(resi_grid_id));return String.valueOf(distance);}
}
get_city_or_prov_id
传入县id,返回city_id或province_id
示例:
返回city_id:
select get_city_or_prov_id(“8340104”,“city”);
返回province_id: select get_city_or_prov_id(“8340104”,“province”);
package ctyun.udf;import ctyun.udf.util.SSXRelation;
import org.apache.hadoop.hive.ql.exec.UDF;public class getCityIdOrProvID extends UDF {public String evaluate(String county_id, String param) {// 根据county_id获取 cityID or provinceIDString id = "-1";if ("city".equals(param)) {id = SSXRelation.COUNTY_CITY.get(county_id);} else if ("province".equals(param)) {id = SSXRelation.COUNTY_PROVINCE.get(county_id);}return id;}
}
涉及到的一些表:
ODS层
ods_oidd
OIDD是采集A接口的信令数据,包括手机在发生业务时的位置信息。OIDD信令类型数据分为三大类,呼叫记录、短信记录和用户位置更新记录。
CREATE EXTERNAL TABLE IF NOT EXISTS ods.ods_oidd(mdn string comment '手机号码' ,start_time string comment '业务开始时间' ,county_id string comment '区县编码' ,longi string comment '经度' ,lati string comment '纬度' ,bsid string comment '基站标识' ,grid_id string comment '网格号' ,biz_type string comment '业务类型' ,event_type string comment '事件类型' ,data_source string comment '数据源'
)
comment 'oidd位置数据表'
PARTITIONED BY (day_id string comment '天分区'
)
ROW FORMAT DELIMITED FIELDS TERMINATED BY '\t'
STORED AS INPUTFORMAT 'org.apache.hadoop.mapred.TextInputFormat' OUTPUTFORMAT 'org.apache.hadoop.hive.ql.io.HiveIgnoreKeyTextOutputFormat'
location '/data/tour/ods/ods_oidd'; // 添加分区
alter table ods.ods_oidd add partition(day_id=20180503);
// 加载数据
load data local inpath '/usr/local/soft/ctyun/ods_oidd/day_id=20180503/*' into table ods.ods_oidd partition(day_id=20180503);
ods_wcdr
WCDR采集网络中ABIS接口的数据,基于业务发生过程中三个扇区的测量信息,通过三角定位法确定用户的位置信息。
CREATE EXTERNAL TABLE IF NOT EXISTS ods.ods_wcdr (mdn string comment '手机号码' ,start_time string comment '业务开始时间' ,county_id string comment '区县编码' ,longi string comment '经度' ,lati string comment '纬度' ,bsid string comment '基站标识' ,grid_id string comment '网格号' ,biz_type string comment '业务类型' ,event_type string comment '事件类型' ,data_source string comment '数据源'
)
comment 'wcdr位置数据表'
PARTITIONED BY (day_id string comment '天分区'
)
ROW FORMAT DELIMITED FIELDS TERMINATED BY '\t'
STORED AS INPUTFORMAT 'org.apache.hadoop.mapred.TextInputFormat' OUTPUTFORMAT 'org.apache.hadoop.hive.ql.io.HiveIgnoreKeyTextOutputFormat'
location '/data/tour/ods/ods_wcdr'; // 添加分区
alter table ods.ods_wcdr add partition(day_id=20180503);
// 加载数据
load data local inpath '/usr/local/soft/ctyun/ods_wcdr/day_id=20180503/*' into table ods.ods_wcdr partition(day_id=20180503);
ods_ddr
当前DDR中只有移动数据详单可以提取基站标识,其他语音,短信,增值等业务没有位置信息,不做为数据融合的基础数据。
CREATE EXTERNAL TABLE IF NOT EXISTS ods.ods_ddr(mdn string comment '手机号码' ,start_time string comment '业务开始时间' ,county_id string comment '区县编码' ,longi string comment '经度' ,lati string comment '纬度' ,bsid string comment '基站标识' ,grid_id string comment '网格号' ,biz_type string comment '业务类型' ,event_type string comment '事件类型' ,data_source string comment '数据源'
)
comment 'ddr位置数据表'
PARTITIONED BY (day_id string comment '天分区'
)
ROW FORMAT DELIMITED FIELDS TERMINATED BY '\t'
STORED AS INPUTFORMAT 'org.apache.hadoop.mapred.TextInputFormat' OUTPUTFORMAT 'org.apache.hadoop.hive.ql.io.HiveIgnoreKeyTextOutputFormat'
location '/data/tour/ods/ods_ddr'; // 添加分区
alter table ods.ods_ddr add partition(day_id=20180503);
// 加载数据
load data local inpath '/usr/local/soft/ctyun/ods_ddr/day_id=20180503/*' into table ods.ods_ddr partition(day_id=20180503);
ods_dpi
移动DPI数据数据采集用户移动用户数据上网时移动核心网和PDSN之间接口的数据。
CREATE EXTERNAL TABLE IF NOT EXISTS ods.ods_dpi(mdn string comment '手机号码' ,start_time string comment '业务开始时间' ,county_id string comment '区县编码' ,longi string comment '经度' ,lati string comment '纬度' ,bsid string comment '基站标识' ,grid_id string comment '网格号' ,biz_type string comment '业务类型' ,event_type string comment '事件类型' ,data_source string comment '数据源'
)
comment 'dpi位置数据表'
PARTITIONED BY (day_id string comment '天分区'
)
ROW FORMAT DELIMITED FIELDS TERMINATED BY '\t'
STORED AS INPUTFORMAT 'org.apache.hadoop.mapred.TextInputFormat' OUTPUTFORMAT 'org.apache.hadoop.hive.ql.io.HiveIgnoreKeyTextOutputFormat'
location '/data/tour/ods/ods_dpi'; // 添加分区
alter table ods.ods_dpi add partition(day_id=20180503);
// 加载数据
load data local inpath '/usr/local/soft/ctyun/ods_dpi/day_id=20180503/*' into table ods.ods_dpi partition(day_id=20180503);
DWD层:
dwd_res_regn_mergelocation_msk_d
在ODS层中,由于数据来源不同,原始位置数据被分成了好几张表加载到了我们的ODS层。
为了方便大家的使用,我们在DWD层做了一张位置数据融合表,在这里,我们将oidd、wcdr、ddr、dpi位置数据汇聚到一张表里面,统一字段名,提升数据质量,这样就有了一张可供大家方便使用的明细表了。
CREATE EXTERNAL TABLE IF NOT EXISTS dwd.dwd_res_regn_mergelocation_msk_d (mdn string comment '手机号码' ,start_time string comment '业务开始时间' ,county_id string comment '区县编码' ,longi string comment '经度' ,lati string comment '纬度' ,bsid string comment '基站标识' ,grid_id string comment '网格号' ,biz_type string comment '业务类型' ,event_type string comment '事件类型' ,data_source string comment '数据源'
)
comment '位置数据融合表'
PARTITIONED BY (day_id string comment '天分区'
)
ROW FORMAT DELIMITED FIELDS TERMINATED BY '\t'
STORED AS TEXTFILE
location '/data/tour/dwd/dwd_res_regn_mergelocation_msk_d'; // 添加分区
alter table dwd.dwd_res_regn_mergelocation_msk_d add partition(day_id=20180503);
mergeSQL
// hive 直接运行速度太慢,可用手动load/put文件方式// 手动load
load data local inpath '/usr/local/soft/ctyun/dwd_merge/part-00000*' into table dwd.dwd_res_regn_mergelocation_msk_d partition(day_id=20180503);// union all
insert into table dwd.dwd_res_regn_mergelocation_msk_d partition(day_id="20180503")
select mdn ,start_time ,county_id ,longi ,lati ,bsid ,grid_id ,biz_type ,event_type ,data_source
from ods.ods_oidd
where day_id = "20180503"
union all
select mdn ,start_time ,county_id ,longi ,lati ,bsid ,grid_id ,biz_type ,event_type ,data_source
from ods.ods_wcdr
where day_id = "20180503"
union all
select mdn ,start_time ,county_id ,longi ,lati ,bsid ,grid_id ,biz_type ,event_type ,data_source
from ods.ods_dpi
where day_id = "20180503"
union all
select mdn ,start_time ,county_id ,longi ,lati ,bsid ,grid_id ,biz_type ,event_type ,data_source
from ods.ods_ddr
where day_id = "20180503";
dwm_staypoint_msk_d
计算一个人在一个网格内的停留时间,按手机号,网格id,区县id分组
1、对所有时间进行排序
2、取第一个点的开始时间和最后一个点的结束时间
CREATE EXTERNAL TABLE IF NOT EXISTS dwm.dwm_staypoint_msk_d (mdn string comment '用户手机号码' ,longi string comment '网格中心点经度' ,lati string comment '网格中心点纬度' ,grid_id string comment '停留点所在电信内部网格号' ,county_id string comment '停留点区县' ,duration string comment '机主在停留点停留的时间长度(分钟),lTime-eTime' ,grid_first_time string comment '网格第一个记录位置点时间(秒级)' ,grid_last_time string comment '网格最后一个记录位置点时间(秒级)'
)
comment '停留点表'
PARTITIONED BY (day_id string comment '天分区'
)
ROW FORMAT DELIMITED FIELDS TERMINATED BY '\t'
STORED AS TEXTFILE
location '/data/tour/dwm/dwm_staypoint_msk_d';
通过grid_id 网格id 获取 网格中心点经纬度 longi、lati
SQL
该SQL执行会出现问题: 执行流程一直处于 0% Map 0% reduce
/**
insert OVERWRITE table dwm.dwm_staypoint_msk_d partition(day_id=20180503)
select t1.mdn,get_points(grid_id)[0] as longi,get_points(grid_id)[1] as lati,t1.grid_id,t1.county_id,dateBetweenUDF(t1.grid_first_time,t1.grid_last_time) as duration,t1.grid_first_time,t1.grid_last_time
from (select mdn,grid_id,county_id,min(split(start_time,',')[0]) as grid_first_time,max(split(start_time,',')[1]) as grid_last_timefrom dwd.dwd_res_regn_mergelocation_msk_dwhere day_id="20180503"group by mdn, grid_id, county_id
)t1;
*/优化后的SQL:WITH split_table as (
SELECT
mdn
,grid_id
,county_id
,split(start_time,',')[1] as grid_first_time
,split(start_time,',')[0] as grid_last_time
FROM dwd.dwd_res_regn_mergelocation_msk_d
where day_id="20180503"
)
, max_min_table as (
SELECT
mdn
,grid_id
,county_id
,Max(grid_first_time) OVER(PARTITION BY mdn,grid_id,county_id) as grid_first_time
,MIN(grid_last_time) OVER(PARTITION BY mdn,grid_id,county_id) as grid_last_time
FROM split_table
)insert OVERWRITE table dwm.dwm_staypoint_msk_d partition(day_id=20180503)
SELECT
t1.mdn
,get_points(t1.grid_id)[0] as longi
,get_points(t1.grid_id)[1] as lati
,t1.grid_id
,t1.county_id
,dateBetweenUDF(t1.grid_first_time,t1.grid_last_time) as duration
,t1.grid_first_time
,t1.grid_last_time
FROM (
SELECT
mdn
,grid_id
,county_id
,grid_first_time
,grid_last_time
FROM max_min_table
group by
mdn
,grid_id
,county_id
,grid_first_time
,grid_last_time
) t1
dws_province_tourist_msk_d
游客定义
出行距离大于300km
常住地在 用户画像表 中
在省内停留时间大于3个小时
CREATE EXTERNAL TABLE IF NOT EXISTS dws.dws_province_tourist_msk_d (mdn string comment '手机号大写MD5加密' ,source_county_id string comment '游客来源区县' ,d_province_id string comment '旅游目的地省代码' ,d_stay_time double comment '游客在该省停留的时间长度(小时)' ,d_max_distance double comment '游客本次出游距离'
)
comment '旅游应用专题数据省级别-天'
PARTITIONED BY (day_id string comment '日分区'
)
ROW FORMAT DELIMITED FIELDS TERMINATED BY '\t'
STORED AS PARQUET
location '/data/tour/dws/dws_province_tourist_msk_d';
停留点表dwm_staypoint_msk_d与用户画像维表dim_usertag_msk_m 通过mdn关联,使用get_city_or_prov_id(county_id,“province”)方法,传入county_id,返回province_id,然后按mdn、province_id、resi_county_id分组,使用calLength(grid_id, resi_grid_id) 传入网格id、居住地网格id,算出出行距离,并计算每个用户到每个省的累计出行时间,然后取出 累计时间最大值超过3小时(180分钟),出行距离大于300km的用户
dws_city_tourist_msk_d
出行距离大于100km
在市内停留时间大于3个小时
CREATE EXTERNAL TABLE IF NOT EXISTS dws.dws_city_tourist_msk_d (mdn string comment '手机号大写MD5加密' ,source_county_id string comment '游客来源区县' ,d_city_id string comment '旅游目的地市代码' ,d_stay_time double comment '游客在该省市停留的时间长度(小时)' ,d_max_distance double comment '游客本次出游距离'
)
comment '旅游应用专题数据城市级别-天'
PARTITIONED BY (day_id string comment '日分区'
)
ROW FORMAT DELIMITED FIELDS TERMINATED BY '\t'
STORED AS PARQUET
location '/data/tour/dws/dws_city_tourist_msk_d';
停留点表dwm_staypoint_msk_d与用户画像维表dim_usertag_msk_m 通过mdn关联,使用get_city_or_prov_id(county_id,“city”)方法,传入county_id,返回city_id,然后按mdn、city_id、resi_county_id分组,使用calLength(grid_id, resi_grid_id) 传入网格id、居住地网格id,算出出行距离,并计算每个用户到每个市的累计出行时间,然后取出 累计时间最大值超过3小时(180分钟),出行距离大于100km的用户
dws_county_tourist_msk_d
出行距离大于10km
在县内停留时间大于3个小时
CREATE EXTERNAL TABLE IF NOT EXISTS dws.dws_county_tourist_msk_d (mdn string comment '手机号大写MD5加密' ,source_county_id string comment '游客来源区县' ,d_county_id string comment '旅游目的地县代码' ,d_stay_time double comment '游客在该县停留的时间长度(小时)' ,d_max_distance double comment '游客本次出游距离'
)
comment '旅游应用专题数据县级别-天'
PARTITIONED BY (day_id string comment '日分区'
)
ROW FORMAT DELIMITED FIELDS TERMINATED BY '\t'
STORED AS PARQUET
location '/data/tour/dws/dws_county_tourist_msk_d';
停留点表dwm_staypoint_msk_d与用户画像维表dim_usertag_msk_m 通过mdn关联,按mdn、county_id、resi_county_id分组,使用calLength(grid_id, resi_grid_id) 传入网格id、居住地id,算出出行距离,并计算每个用户到每个县的累计出行时间,然后取出 累计时间最大值超过3小时(180分钟),出行距离大于10km的用户
SQL
insert into table dws.dws_county_tourist_msk_d partition(day_id="20180503")
select ttt1.mdn,ttt1.source_county_id,ttt1.d_county_id,ttt1.d_stay_time,ttt1.d_max_distance
from(select mdn,resi_county_id as source_county_id,county_id as d_county_id,sum(duration) as d_stay_time,max(calLength(tt1.grid_id,tt1.resi_grid_id)) as d_max_distancefrom(select t1.mdn,t1.grid_id,t1.county_id,t1.duration,t2.resi_county_id,t2.resi_grid_idfrom (select *from dwm.dwm_staypoint_msk_dwhere day_id='20180503') t1 join(select *from dim.dim_usertag_msk_mwhere month_id='201805') t2 on t1.mdn = t2.mdn) tt1 group by tt1.mdn,tt1.county_id,tt1.resi_county_id
)ttt1 where d_stay_time > 180 and d_max_distance > 10000
;
DIM层
dim_usertag_msk_m
CREATE EXTERNAL TABLE IF NOT EXISTS dim.dim_usertag_msk_m (mdn string comment '手机号大写MD5加密' ,name string comment '姓名' ,gender string comment '性别,1男2女' ,age string comment '年龄' ,id_number string comment '证件号码' ,number_attr string comment '号码归属地' ,trmnl_brand string comment '终端品牌' ,trmnl_price string comment '终端价格',packg string comment '套餐' ,conpot string comment '消费潜力' ,resi_grid_id string comment '常住地网格' ,resi_county_id string comment '常住地区县'
)
comment '用户画像表'
PARTITIONED BY (month_id string comment '月分区'
)
ROW FORMAT DELIMITED FIELDS TERMINATED BY '\t'
STORED AS PARQUET
location '/data/tour/dim/dim_usertag_msk_m'; // 添加分区
alter table dim.dim_usertag_msk_m add partition(month_id=201805);
// 加载数据
load data local inpath '/usr/local/soft/ctyun/dim_usertag_msk_m/month_id=201805/*' into table dim.dim_usertag_msk_m partition(month_id=201805);
ADS层
根据需求建设
需求矩阵
根据省游客表计算如下指标
-
客流量按天 [省id,客流量]
-
性别按天 [省id,性别,客流量]
-
年龄按天 [省id,年龄,客流量]
-
常住地按天 [省id,常住地市,客流量]
-
归属地按天 [省id,归属地市,客流量]
-
终端型号按天 [省id,终端型号,客流量]
-
消费等级按天 [省id,消费等级,客流量]
-
停留时长按天 [省id,停留时长,客流量]
根据市游客表计算如下指标
- 客流量按天 [市id,客流量]
- 性别按天 [市id,性别,客流量]
- 年龄按天 [市id,年龄,客流量]
- 常住地按天 [市id,常住地市,客流量]
- 归属地按天 [市id,归属地市,客流量]
- 终端型号按天 [市id,终端型号,客流量]
- 消费等级按天 [市id,消费等级,客流量]
- 停留时长按天 [市id,停留时长,客流量]
根据区县游客表计算如下指标
- 客流量按天 [区县id,客流量]
select t1.d_county_id,count(*) as d_county_cnt
from (select d_county_idfrom dws.dws_county_tourist_msk_dwhere day_id="20180503"
)t1 group by t1.d_county_id;
- 性别按天 [区县id,性别,客流量]
select t1.d_county_id,t2.gender,count(*) as d_county_gender_cnt
from(select mdn,d_county_idfrom dws.dws_county_tourist_msk_dwhere day_id="20180503"
) t1 left join (select mdn,genderfrom dim.dim_usertag_msk_mwhere month_id=201805
) t2 on t1.mdn = t2.mdn
group by t1.d_county_id,t2.gender;
- 年龄按天 [区县id,年龄,客流量]
- 常住地按天 [区县id,常住地市,客流量]
- 归属地按天 [区县id,归属地市,客流量]
select t1.d_county_id,t2.number_attr,count(*) as d_county_number_attr_cnt
from(select mdn,d_county_idfrom dws.dws_county_tourist_msk_dwhere day_id="20180503"
) t1 left join (select mdn,number_attrfrom dim.dim_usertag_msk_mwhere month_id=20180503
) t2 on t1.mdn = t2.mdn
group by t1.d_county_id,t2.number_attr;
- 终端型号按天 [区县id,终端型号,客流量]
- 消费等级按天 [区县id,消费等级,客流量]
- 停留时长按天 [区县id,停留时长,客流量]